|
|
About the Conference |
|
|
|
|
|
|
Conference Program |
|
|
|
|
|
|
|
|
|
|
|
|
Venue and Travel |
|
|
|
|
|
|
|
|
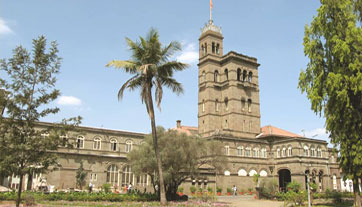 |
COMAD is organized by Division II and
Pune Chapter of Computer Society of India (CSI)
and Special Interest Group on Data (SIGDATA). |
|
|
|
Invited Industry Talks |
|
Reinventing the Technology Stack at Oracle Labs:
Nipun Agarwal, Oracle
For the last 40 years, the technology stack for computing (hardware, OS, databases, languages & compilers) have been built around a similar sets of interfaces that are locked into place by backwards-compatibility requirements. At Oracle Labs, we have a set of projects that are looking to reinvent this stack with the understanding that innovation at one level requires adaptation at the other levels. For example, one is very limited in hardware innovation if you must keep all existing software running with no performance regressions. The era of big data and cloud computing provides us with an opportunity to change these interfaces to provide an order of magnitude of performance improvements in many of the tech stack components.
Presenter Bio : Nipun Agarwal is Vice President of database research and software advanced development at Oracle Labs and is based in Redwood Shores, California. Prior to this role, he held various senior technical and management positions in the Oracle database group and has been awarded over 125 patents. He is directing a number of research initiatives at Oracle with the aim of transferring these technologies into Oracle products. The research and advanced development spans a broad range of technologies ranging from databases designed for massively parallel architectures to ultra low latency processing for network packets. Nipun is also responsible for business innovation to introduce these cutting edge technologies in the market which can substantially improve customers business. |
|
|
|
Entity Mining at Microsoft Bing
Manish Gupta, Microsoft
Entity mining is a hot area of research. At Microsoft Bing, we perform a large number of entity mining tasks which continuously populate and use Bing's knowledge graph, Satori. In this talk, I will discuss a few of such interesting tasks:
(1) entity linking in the Microsoft Edge and Snapshots on Tap, (2) extracting fictional character entities from books,
(3) extracting disaster event entities from Twitter, and (4) event entity linking for sports events.
Presenter bio: Manish Gupta is a Senior Applied Scientist at the Bing team in Microsoft India R&D Private Limited at Hyderabad, India. He is also an Adjunct Faculty at International Institute of Information Technology, Hyderabad. He received his Masters in Computer Science from IIT Bombay in 2007 and his Ph.D. from the University of Illinois at Urbana-Champaign in 2013. Before this, he worked for Yahoo! Bangalore for two years. His research interests are in the areas of web mining, data mining and information retrieval. He has published more than 40 research papers in reputed referred journals and conferences. He has also co-authored two books: one on Outlier Detection for Temporal Data and another one on Information Retrieval with Verbose Queries. |
|
|
|
Introduction to Knowledge Graph Stores with Applications
Sameep Mehta, IBM Research
This talk will give an introduction to popular Graph Stores like Titan, Neo4J, etc. We will motivate the applications for which graphs present a natural modeling choices. We will discuss popular triplet stores like RDFs and how they compare and contrast with graph stores. The talk will argue on the scalability aspect of the data and show how the volume of data is handled by graph stores at back end. The attendees will be exposed to common graph querying language and search capabilities including elastic search. The talk will focus on one end-to-end scenario to show different steps like data preparation, data ingestion and accessing the data through APIs. We will conclude the data to by showing a demo of application built on graphs.
Presenter bio: Sameep Mehta is a Senior Researcher and Manager at IBM Research - India. He received his Ph.D. in Data Mining and Visualization from Ohio State University. His current research interests are Data Mining, Text Mining, Machine Learning, Big Data Technologies, Social Data Analytics and Knowledge Graph. He has published extensively in top conferences in Data Mining, Services and Visualization. He is a regular speaker at conferences and is PC chair for Big Data Analytics Conference 2014. He also serves as Adjunct Faculty at IIIT-Delhi in the area of Data Analytics. |
|
|
|
Big Data @ Flipkart: Driving Intelligence at Scale
Gaurav Bhalotia, Flipkart
Data is the only true IP for an internet company, even more than scale and infrastructure. Over the past few years, there has been a strong trend in the industry around data and products that use data to make intelligent decisions. At Flipkart we have the largest amount of commerce data in the country with 5TB data getting added everyday. In this talk I will share our approach to data and how we are building realtime systemic intelligence to power great experiences for our users. I will motivate the need for a central infrastructure that provides large scale, reliable storage, processing and querying of this data. I will walk through the high level architecture of our data platform and some interesting technology challenges that we encounter. If time permits I will delve deeper into the details of our query engine 'Apache Lens' which recently graduated into a top level Apache project.
Presenter bio: Gaurav Bhalotia is Vice President (Engineering) and heads Data Platform at Flipkart. He has nearly 13 years of experience architecting, implementing and leading development of large-scale distributed systems. He was director of Engineering at Kosmix, where he led the development of a local search engine and also created the first version of their categorization engine.
Gaurav is a graduate of Indian Institute of Technology Mumbai and has a MS in Computer Science from University of California Berkeley. He has filed multiple patents and has several conference publications in Search, Categorization and Information Extraction. |
|
|
|
ShareInsights: An Unified Approach to Full-stack Data Processing
Mukund Deshpande, Persistent Systems
The field of data analysis seeks to extract value from data for either business or scientific benefit. This field has seen a renewed interest with the advent of big data technologies and a new organizational role called data scientist. Even with the new found focus, the task of analyzing large amounts of data is still challenging and time-consuming.
The essence of data analysis involves setting up data pipe-lines which consists of several operations that are chained together - starting from data collection, data quality checks, data integration, data analysis and data visualization (including the setting up of interaction paths in that visualization).
In our opinion, the challenges stem from from the technology diversity at each stage of the data pipeline as well as the lack of process around the analysis.
In this paper we present a platform that aims to significantly reduce the time it takes to build data pipelines. The platform attempts to achieve this in following ways.
- Allow the user to describe the entire data pipeline with a single language and idioms - all the way from data ingestion to insight expression (via visualization and end-user interaction).
- Provide a rich library of parts that allow users to quickly assemble a data analysis pipeline in the language.
- Allow for a collaboration model that allows multiple users to work together on a data analysis pipeline as well as leverage and extend prior work with minimal effort.
We studied the efficacy of the platform for a data hackathon competition conducted in our organization. The hackathon provided us with a way to study the impact of the approach. Rich data pipelines which traditionally took weeks to build were constructed and deployed in hours. Consequently, we believe that the complexity of designing and running the data analysis pipeline can be significantly reduced; leading to a marked improvement in the productivity of data analysts/data scientists. |
|
|
|
|
|