|
|
Conference Program |
|
About the Conference |
|
Venue and Travel |
|
WBDB |
|
|
|
|
|
Keynote Talks |
|
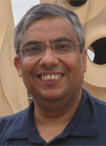 |
Dr. Rakesh Agrawal is a Microsoft Technical Fellow, heading the Search Labs in Microsoft Research in Silicon Valley.
Rakesh is a Member of the National Academy of Engineering, a Fellow of ACM, and a Fellow of IEEE. He is the recipient of the 2010 IIT-Rookee Distinguished Alumni Award, ACM-SIGKDD First Innovation Award, ACM-SIGMOD Edgar F. Codd Innovations Award, ACM-SIGMOD Test of Time Award, VLDB 10-Yr Most Influential Paper Award, and the Computerworld First Horizon Award. Scientific American named him to the list of 50 top scientists and technologists in 2003. |
|
|
Rakesh is a Member of the National Academy of Engineering, a Fellow of ACM, and a Fellow of IEEE. He is the recipient of the 2010 IIT-Rookee Distinguished Alumni Award, ACM-SIGKDD First Innovation Award, ACM-SIGMOD Edgar F. Codd Innovations Award, ACM-SIGMOD Test of Time Award, VLDB 10-Yr Most Influential Paper Award, and the Computerworld First Horizon Award. Scientific American named him to the list of 50 top scientists and technologists in 2003. |
|
Before joining Microsoft in March 2006, Rakesh worked as an IBM Fellow at the IBM Almaden Research Center. Earlier, he was with the Bell Laboratories, Murray Hill from 1983 to 1989. He also worked for three years at the Bharat Heavy Electricals Ltd. in India. He received the M.S. and Ph.D. degrees in Computer Science from the University of Wisconsin-Madison in 1983. He also holds a B.E. degree in Electronics and Communication Engineering from IIT-Roorkee, and a two-year Post Graduate Diploma in Industrial Engineering from the National Institute of Industrial Engineering (NITIE), Bombay. |
|
Title: Reimagining Textbooks Through the Data Lens |
|
Abstract: Education is known to be the key determinant of economic growth and prosperity [8, 12]. While the issues in devising a high-quality educational system are multi-faceted and complex, textbooks are acknowledged to be the educational input most consistently associated with gains in student learning [11]. They are the primary conduits for delivering content knowledge to the students and the teachers base their lesson plans primarily on the material given in textbooks [7].
With the emergence of abundant online content, cloud computing, and electronic reading devices, textbooks are poised for transformative changes. Notwithstanding understandable misgivings (e.g. Gutenberg Elegies [6]), textbooks cannot escape what Walter Ong calls ‘the technologizing of the word’ [9]. The electronic format comes naturally to the current generation of ‘digital natives’ [10]. Inspired by the emergence of this new medium for “printing” and “distributing” textbooks, we present our early explorations into developing a data mining based approach for enhancing the quality of electronic textbooks. Specifically, we first describe a diagnostic tool for authors and educators to algorithmically identify deficiencies in textbooks. We then discuss techniques for algorithmically augmenting different sections of a book with links to selective content mined from the Web.
Our tool for diagnosing deficiencies consists of two components. Abstracting from the education literature, we identify the following properties of good textbooks: (1) Focus : Each section explains few concepts, (2) Unity: For every concept, there is a unique section that best explains the concept, and (3) Sequentiality: Concepts are discussed in a sequential fashion so that a concept is explained prior to occurrences of this concept or any related concept. Further, the tie for precedence in presentation between two mutually related concepts is broken in favor of the more significant of the two. The first component provides an assessment of the extent to which these properties are followed in a textbook and quantifies the comprehension load that a textbook imposes on the reader due to non-sequential presentation of concepts [1, 2]. The second component identifies sections that are not written well and can benefit from further exposition. We propose a probabilistic decision model for this purpose, which is based on the syntactic complexity of writing and the notion of the dispersion of key concepts mentioned in the section [4].
For augmenting a section of a textbook, we first identify the set of key concept phrases contained in a section. Using these phrases, we find web articles that represent the central concepts presented in the section and endow the section with links to them [5]. We 13th Internationl Conference on Management of Data (COMAD) Pune, India, December 2012. also describe techniques for finding images that are most relevant to a section of the textbook, while respecting the constraint that the
same image is not repeated in different sections of the same chapter. We pose this problem of matching images to sections in a textbook chapter as an optimization problem and present an efficient algorithm for solving it [3].
We finally provide the results of applying the proposed techniques to a corpus of widely-used, high school textbooks published
by the National Council of Educational Research and Training (NCERT), India. We consider books from grades IX–XII, covering four broad subject areas, namely, Sciences, Social Sciences, Commerce, and Mathematics. The preliminary results are encouraging and indicate that developing technological approaches to embellishing text-books could be a promising direction for research. |
|
1. REFERENCES |
[1] R. Agrawal, S. Chakraborty, S. Gollapudi, A. Kannan, and K. Kenthapadi. Empowering authors to diagnose comprehension burden in textbooks. In KDD, 2012.
[2] R. Agrawal, S. Chakraborty, S. Gollapudi, A. Kannan, and K. Kenthapadi. Quality of textbooks: An empirical study. In ACM DEV, 2012.
[3] R. Agrawal, S. Gollapudi, A. Kannan, and K. Kenthapadi. Enriching textbooks with images. In CIKM, 2011.
[4] R. Agrawal, S. Gollapudi, A. Kannan, and K. Kenthapadi. Identifying enrichment candidates in textbooks. In WWW, 2011.
[5] R. Agrawal, S. Gollapudi, K. Kenthapadi, N. Srivastava, and R. Velu. Enriching textbooks through data mining. In ACM DEV, 2010.
[6] S. Birkerts. The Gutenberg Elegies: The Fate of Reading in an Electronic Age. Faber & Faber, 2006.
[7] J. Gillies and J. Quijada. Opportunity to learn: A high impact strategy for improving educational outcomes in developing countries. USAID Educational Quality Improvement Program (EQUIP2), 2008.
[8] E. A. Hanushek and L. Woessmann. The role of education quality for economic growth. Policy Research Department Working Paper 4122, World Bank, 2007.
[9] W. J. Ong. Orality & Literacy: The Technologizing of the Word. Methuen, 1982.
[10] M. Prensky. Digital natives, digital immigrants. On the Horizon, 9(5), 2001.
[11] A. Verspoor and K. B. Wu. Textbooks and educational development. Technical report, World Bank, 1990.
[12] World-Bank. Knowledge for Development: World Development Report: 1998/99. Oxford, 1999. |
|
|
|
|
|
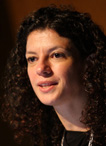 |
Sihem Amer-Yahia is Principal Research Scientist at Qatar ComputingResearch Center (QCRI) and DR1 CNRS at LIG in Grenoble. Sihem's interests are at the intersection of large-scale data management and analytics, and social content at large. Until May 2011, she was Senior Scientist at Yahoo! Research for 5 years and worked on revisiting relevance models and top-k processing algorithms on datasets from Delicious, Yahoo! Personals and Flickr. Before that, she spent 7 years at AT&T Labs in NJ, working on XML query optimization and XML full-text search. Sihem is editor of the W3C XML full-text standard. She is a member of the VLDB Endowment and the ACM SIGMOD executive committee. Sihem is track chair at PVLDB and SIGIR this
year. She serves on the editorial boards of ACM TODS, the VLDB Journal and the Information Systems |
|
Journal. Sihem received her Ph.D. in Computer Science from Univ. Paris-Orsay and INRIA in 1999, and her Diplome d’Ingenieur from INI, Algeria in 1994. |
|
Title: User Activity Analytics on the Social Web of News |
|
Abstract: The proliferation of social media is undoubtedly changing the way people produce and consume news online. Editors and publishers in newsrooms need to understand user engagement and audience sentiment evolution on various news topics. News consumers want to explore public reaction on articles relevant to a topic and refine their exploration via related entities, topics, articles and tweets. I will present MAQSA, a system for social analytics on news. MAQSA provides an interactive topic-centric dashboard that summarizes social activity around news articles. The dashboard contains an annotated comment timeline, a social graph of comments, and maps of comment sentiment and topics. The analysis of both content and user engagement in social media in MAQSA enables the exploration of new ways of immersing users in a news consumption experience. |
|
|
|
|
|
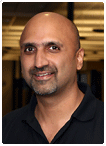 |
Rajeev Rastogi is the Director of Machine Learning at Amazon. Previously, he was the Vice President of Yahoo! Labs Bangalore, and a Bell Labs Fellow at Bell Labs in Murray Hill, NJ. Rajeev is active in the fields of databases, data mining, and networking, and has served on the program committees of several conferences in these areas. He currently serves on the editorial board of the CACM, and has been an Associate editor for IEEE Transactions on Knowledge and Data Engineering in the past. He has published over 125 papers, and holds over 50 patents. Rajeev received his B. Tech degree from IIT Bombay, and a PhD degree in Computer Science from the University of Texas, Austin. |
|
|
Title: Building knowledge bases from the web |
|
Abstract: The web is a vast repository of human knowledge. Extracting structured data from web pages can enable applications like comparison shopping, and lead to improved ranking and rendering of search results. In this talk, I will describe two efforts to extract records from pages at web scale. The first is a wrapper induction system that handles end-to-end extraction tasks from clustering web pages to learning XPath extraction rules to relearning rules when sites change. The system has been deployed in production within Yahoo! to extract more than 500 million records from ~200 web sites. The second effort exploits machine learning models to automatically extract records without human supervision. Specifically, we use Markov Logic Networks (MLNs) to capture content and structural features in a single unified framework, and devise a fast graph-based approach for MLN inference. |
|
|
|
|
|